
|
 |
Back to 2018 Program and Abstracts
MACHINE LEARNING ANALYSIS OF THE WHOLE RECTAL WALL ON POST-NEOADJUVANT CHEMORADIATION MRI MAY OFFER ACCURATE IDENTIFICTION OF RECTAL CANCER PATIENTS NEEDING MORE AGGRESSIVE FOLLOW-UP OR SURGERY
Jacob Antunes*1, Amrish Selvam1, Kaustav Bera1, Justin Brady2, Joseph Willis3, Raj mohan Paspulati4, Anant Madabhushi1, Conor P. Delaney5, Satish Viswanath1 1Biomedical Engineering, Case Western Reserve University, Cincinnati, OH; 2Surgery, University Hospitals Cleveland Medical Center, Cleveland, OH; 3Pathology, University Hospitals Cleveland Medical Center, Cleveland, OH; 4Radiology, University Hospitals Cleveland Medical Center, Cleveland, OH; 5Digestive Disease and Surgery Institute, Cleveland Clinic, Cleveland, OH
BACKGROUND: A critical question in rectal cancers is in vivo evaluation of tumor regression after neoadjuvant chemoradiation for surgical planning. However, it is difficult to definitively identify viable tumor regions on routine post-chemoradiation magnetic resonance imaging (MRI). Further, tumor involvement with the adjacent rectal wall is not visually distinctive on MRI, and has not been widely explored. Our aim was to apply machine learning to computer-extracted image appearance (radiomic) features of the rectal wall on post-chemoradiation MRIs, to segregate patients who need aggressive surgery and follow-up from those who only need a conventional total mesorectal excision (TME). METHODS: This single-center, retrospective study utilized pre-operative, post-chemoradiation MRIs from rectal cancer patients who underwent neoadjuvant chemoradiation followed by surgery between 2009 and 2015. An expert radiologist annotated (1) tumor-suspicious, and (2) rectum adjacent to tumor (RATT); within the rectal wall on all MRIs. 123 pixel-wise radiomic features were calculated from each of (a) tumor, (b) RATT, and (c) the entire rectal wall; yielding texture-based measurements of intensity and gradient heterogeneity within these regions of interest (ROIs). Statistical testing and random forest-based machine learning were used to build predictors based on each of the 3 ROIs to discriminate patients only needing conventional TME from patients requiring more extensive surgery or follow-up. Predictors were evaluated using receiver-operator-characteristic (ROC) curve analysis, and performance quantified via the area under the ROC curve (AUC). RESULTS: 36 patients (15 females) were included with a mean age of 65.6 years at time of post-treatment MRI. 20 patients only required TME and 16 patients needed more extensive surgery or adjuvant treatment. The radiomics predictor based on the whole rectal wall yielded the best performance in distinguishing patients needing TMEs from those needing more aggressive surgery/follow-up (AUC=0.74±0.09). By comparison, the tumor radiomic predictor (AUC=0.62±0.06) and RATT predictor (AUC=0.66±0.07) demonstrated only moderate performance. Additionally, MRI intensities within each of the 3 ROIs showed no significant differences between patient groups. The most relevant radiomic features within the whole rectal wall ROI comprised gradient and ripple/wave heterogeneity patterns, indicating presence of sub-visual tumor- infiltration characteristics both within and around the tumor region. CONCLUSIONS: Computer-extracted tissue heterogeneity descriptors of the whole rectal wall on rectal MRIs could be used to build novel machine learning-based predictive tools for early, non-invasive identification of rectal cancer patients needing more aggressive surgery in order to optimally personalize their follow-up.
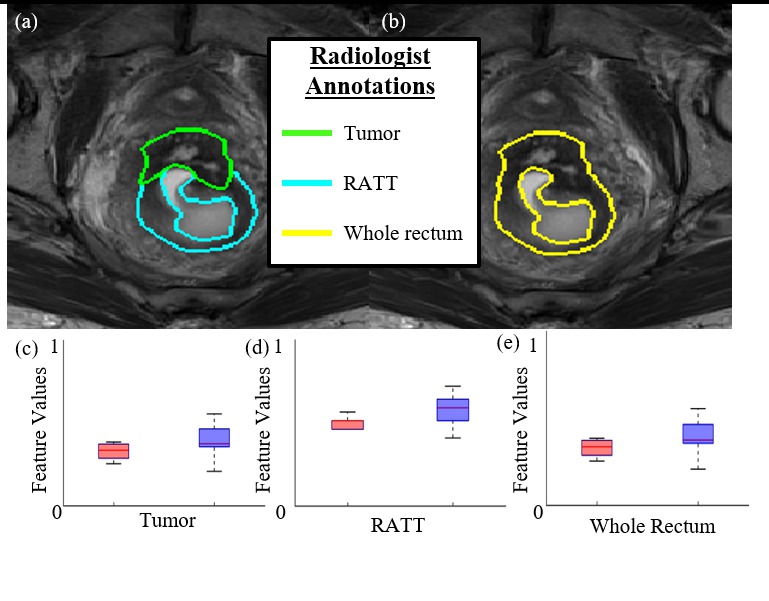 Figure 1: (a) T2w MRI of a single patient with corresponding expert radiologist annotations of tumor (green) and RATT (cyan). (b) The union of tumor and RATT annotations to comprise the entire rectal wall (in yellow). (c,d,e) Boxplots of normalized radiomic feature values extracted within each region of interest, showing markedly different expressions between patient groups. Optimal separation of patient groups and predictive performance was achieved via radiomic features derived from the whole rectum ROI.
Back to 2018 Program and Abstracts
|